A new machine-learning algorithm developed by the U.S. Department of Energy’s Pacific Northwest National Laboratory could help urban transportation analysts relieve bottlenecks and chokepoints that routinely snarl city traffic.
[Above image via PNNL.]
The new tool – called TranSEC, which stands for “Transportation State Estimation Capability” – uses traffic datasets collected from Uber drivers and other publicly available traffic sensor data to map street-level traffic flow over time.
It then uses machine learning to connect known information segments with missing data, allowing the program to make near real-time street level estimations, explained Arif Khan, a PNNL computer scientist who helped develop TranSEC.
“What’s novel here is the street level estimation over a large metropolitan area,” he explained in a statement. “Unlike other models that only work in one specific metro area, our tool is portable and can be applied to any urban area where aggregated traffic data is available.”
Using public data from the entire 1,500-square-mile Los Angeles metropolitan area, the PNNL team reduced the time needed to create a traffic congestion model by an order of magnitude – from hours down to mere minutes. That speed-up, accomplished with high-performance computing resources at PNNL, makes near-real-time traffic analysis feasible.
The machine-learning feature of TranSEC means that, as it acquires and processes more data, the system becomes more refined and useful over time – helping understand how “disturbances” spread across traffic networks. Given enough data, the machine-learning element will be able to predict impacts so that traffic engineers can create corrective strategies, PNNL said.
“We use a graph-based model together with novel sampling methods and optimization engines, to learn both the travel times and the routes,” added Arun Sathanur, a PNNL computer scientist and a lead researcher on the team. “The method has significant potential to be expanded to other modes of transportation, such as transit and freight traffic. As an analytic tool, it is capable of investigating how a traffic condition spreads.”
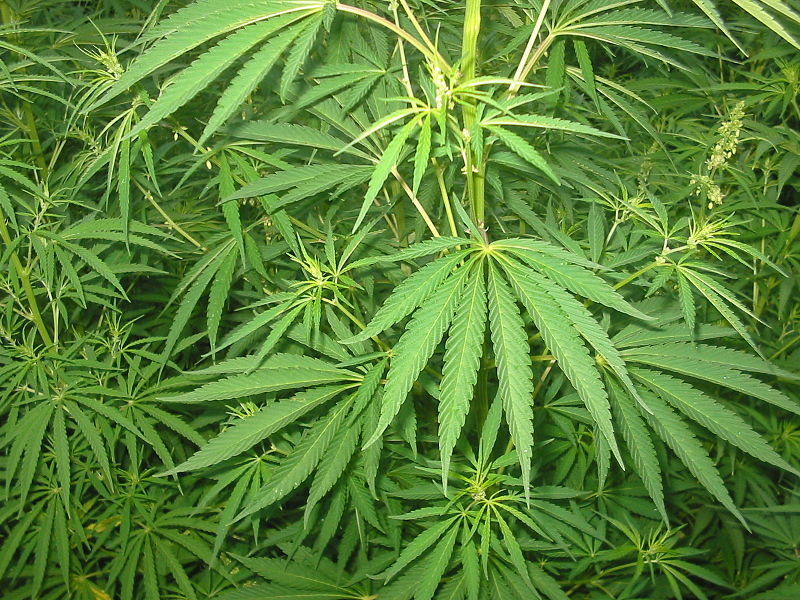